
After some reflection, you are 50 percent confident that \(\mu\) falls between 8 and 12 inches. Suppose you focus on the quantity \(\mu\), the average snowfall during the month of January. You currently live on the west coast of the United States where the weather is warm and you are wondering about the snowfall you will encounter in Buffalo in the following winter season. Suppose you are planning to move to Buffalo, New York. The following sections illustrate this general problem where integrals of the product of the likelihood and prior can not be evaluated analytically and so there are challenges in summarizing the posterior distribution. \pi(\theta \mid y) = \fracĬomputation of the posterior mean requires the evaluation of two integrals, each not expressible in closed-form. In a general Bayesian problem, the data \(Y\) comes from a sampling density \(f(y \mid \theta)\) and the parameter \(\theta\) is assigned a prior density \(\pi(\theta)\).Īfter \(Y = y\) has been observed, the likelihood function is equal to \(L(\theta) = f(y \mid \theta)\) and the posterior density is written as For example, if the posterior density has a Normal form, one uses the R functions pnorm() and qnorm() to compute posterior probabilities and quantiles. In these cases, the posterior distribution has a convenient functional form such as a Beta density or Normal density, and the posterior distributions are easy to summarize. The Bayesian models in Chapters 7 and 8 describe the application of conjugate priors where the prior and posterior belong to the same family of distributions. 13.4.3 Disputed authorship of the Federalist Papers.13.4.2 A latent class model with two classes.13.3.5 Estimating many trajectories by a hierarchical model.13.3.2 Measuring hitting performance in baseball.13.2.6 Which words distinguish the two authors?.13.2.5 Comparison of rates for two authors.12.2 Bayesian Multiple Linear Regression.12 Bayesian Multiple Regression and Logistic Models.11.7 Bayesian Inferences with Simple Linear Regression.11.2 Example: Prices and Areas of House Sales.10.3 Hierarchical Beta-Binomial Modeling.9.6.1 Burn-in, starting values, and multiple chains.9.5.3 Normal sampling – both parameters unknown.9.4.1 Choice of starting value and proposal region.9.3.3 A general function for the Metropolis algorithm.9.3.1 Example: Walking on a number line.9 Simulation by Markov Chain Monte Carlo.8.8.4 Case study: Learning about website counts.8.6.1 Bayesian hypothesis testing and credible interval.8.6 Bayesian Inferences for Continuous Normal Mean.
#QUCS SIMULATION SHARP TRANSITIONS TROUBLE UPDATE#
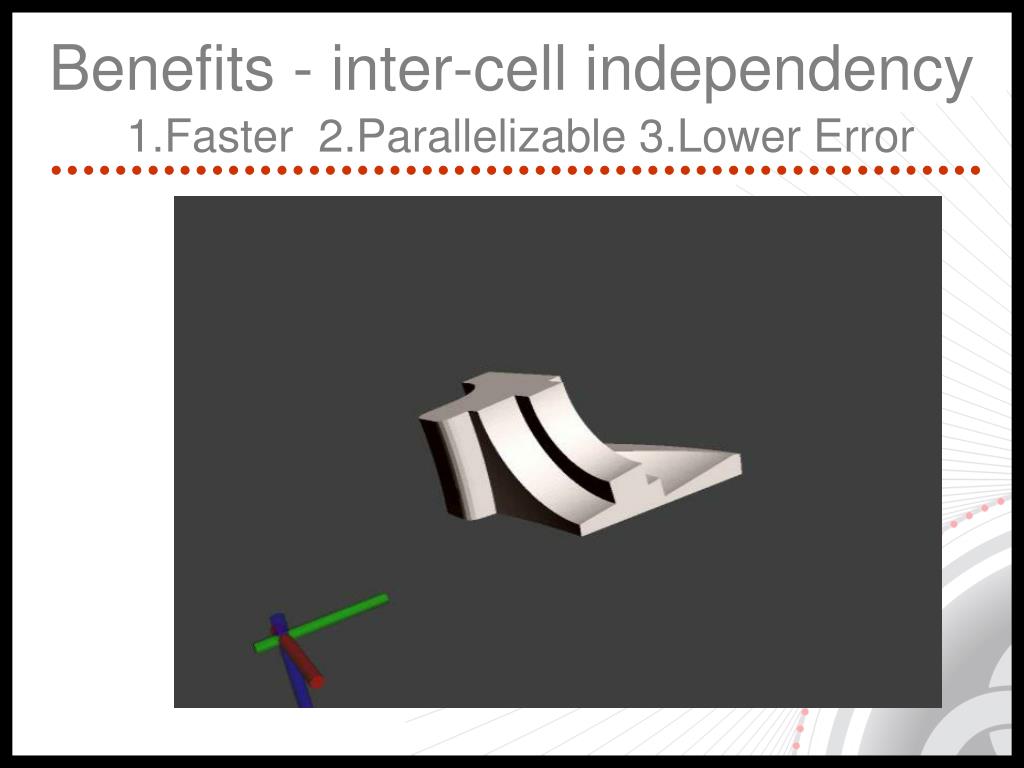
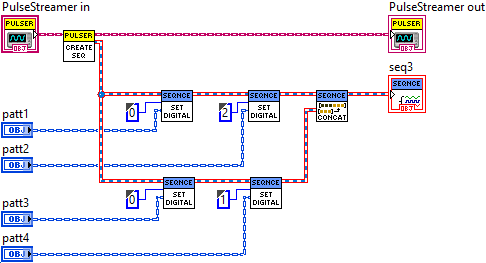
